Throwing out the rulebook: How age, race and gender are key inputs for fair lending
October 28, 2024
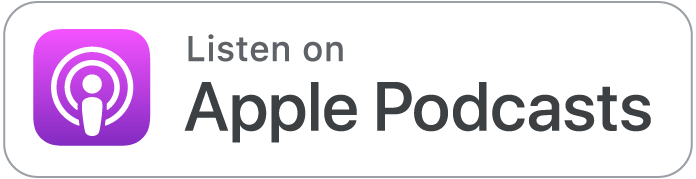
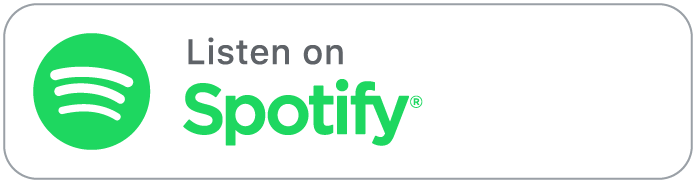
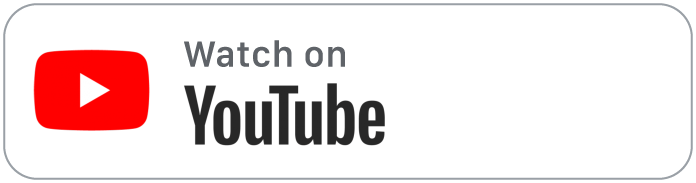
Episode Summary
In this first of two episodes with Kareem Saleh, Founder and CEO of FairPlay AI, host Vince Passione takes a deep dive into the world of AI underwriting: How it’s been used in the past, how it has fallen short of its potential to remove bias in lending decisioning, and how the pioneering concept of Fairness-as-a-Service may yet revolutionize the AI lending game.
Key takeaways:
2:06 How the murder of George Floyd compelled Kareem Saleh to look for ways he could more effectively change the system he operates in, and increase fairness for everyone.
3:08 Machine learning is capable of learning, but also of learning the wrong things.
3:55 Financial services regulators have clearly defined definitions of “fairness”: One focusing on disparate treatment, the other focusing on disparate impact.
5:18 One of the core challenges (and/or failures to-date) for using AI to mitigate bias is that it can only learn from the data it is trained on; and that typically over-represents certain populations.
8:49 Traditional logistic regression models don’t always work because they assume credit behaviors are linear. In reality, an individual’s financial behaviors are non-linear.
9:31 Unfairness can be born out of AI-driven models when seemingly independent variables interact with one another in a way that most humans wouldn’t interpret, but machines do.
10:01 Underwriting regulation and compliance hasn’t yet caught up with the technological capabilities of our AI-driven world.
11:56 Why monitoring an AI model’s fairness trends is critical.
14:40 Examples of ways seemingly disparate or arbitrary variables can interact with one another to bias an outcome–and how personal attributes are still being leveraged to a greater or lesser extent in decisioning models.
19:53 Concerns vs. regulatory comfort with using personal attributes like age and gender in decisioning.
22:44 The privacy implications, particularly in light of 1033, and how finding a way to share your personal information in some way–even if it’s just through the census–will help contribute to a fairer credit system.
Resources Mentioned:
- https://fairplay.ai/ Fairplay AI
- https://www.fdic.gov/system/files/2024-06/iv-1-1.pdf Fair lending rules and regulations
- https://www.justice.gov/crt/equal-credit-opportunity-act-3 Equal Credit Opportunity Act
- https://www.justice.gov/crt/fair-housing-act-1 Fair Housing Act
- https://www.lendkey.com/podcast/modern-credit-reporting-can-old-tech-handle-new-data/ Christian Widhalm, Bloom Credit, episode
In this episode
Episode Transcript
[00:00] Kareem Saleh: AI has this tendency to overfit its decisions on populations in the data that are well-represented. Populations in the data whose information is present and correct. And the problem, of course, is that for all kinds of historical reasons, there are many populations for whom the data is messy, missing or wrong.
[00:30] Narrator: Welcome to 22 Minutes in Lending, your go-to podcast for insights on all things lending, from lending practices, regulatory updates, how to enhance lending efforts and more. In each episode, Vince Passione connects with industry leaders to discuss the latest trends and happenings around the lending industry. Let’s dive in to the latest in lending.
[00:54] Vince Passione: Welcome, everyone, to 22 Minutes in Lending. I’m your host, Vince Passione, and our guest today is Kareem Saleh, a trailblazer in the fintech industry and a passionate advocate for fairness in AI-driven financial services. As the founder and CEO of FairPlay, Kareem is pioneering the concept of fairness as a service using cutting-edge AI technology-reduced bias and lending and financial decision-making. With an impressive background that includes executive roles at Zest AI and Softcard, as well as key positions in the Obama administration, Kareem brings a wealth of experience in AI, lending and public policy to the table. Thank you Kareem for being on the show, and welcome to 22 Minutes in Lending.
[01:28] Kareem Saleh: Thanks for having me, Vince. I’m delighted to be here.
[01:30] Vince Passione: Great. Well, Kareem, I always do this to all of our entrepreneurs. I want to find out sort of why, the why. So my research, FairPlay was founded in 2020. I took a look at Crunchbase, looks like you guys raised about 14 and a half million dollars in your series A. I know that Nika was one of your investors as well as TTV, that’s also an investor in LendKey. And I took a look at the mission, and what I read on the website was, “The mission is to build fairness infrastructure for the internet so that any company using an algorithm to make high-stakes decisions about people’s lives can ensure those decisions are made fairly.” So why this mission, and why now?
[02:06] Kareem Saleh: Yeah. Well, I think everyone from all walks of life knows what it’s like to be treated unfairly, and the deep and lasting pain that unfairness can cause in people’s lives. In fact, there’s recently been a study that shows that when people see unfairness, the part of the brain that alights is the part of the brain that’s associated with disgust. And so I watched George Floyd’s murder in 2020, and it’s nine minutes of inhumanity, which if you haven’t seen it, will affect you deeply. And his murder I think prompted us, like a lot of people, to ask what can I do to change, to improve the system that I operate in? The system that I have influence? And for us, it was a personal call to action to kind of drive change in the domain that we operate in, which is financial services.
[03:08] Kareem Saleh: And my co-founder and I were early to the application of AI in lending, and we knew that AI is extremely powerful, has the potential to increase approval rates, increase take rates, but also machine learning is capable of learning the wrong things. And I’ve got some funny stories to tell you about that sometime. But because AI is taking over more and more decisions in our lives, the risk of unfairness in AI decisions could deny people jobs, deny people homes, deny people healthcare, deny people government services and deny people financial services. And so we founded the company and our mission, as you say, is to de-bias digital decisions.
[03:56] Vince Passione: So you talk about fairness in service, but what is fairness? How do you define fairness in that realm?
[04:04] Kareem Saleh: Yeah, it’s a great question. So there are many different definitions of fairness, something like 21 at least that I know of. And the difficulty is that many of them conflict with one another. But for those of us who work in financial services, we have the benefit of a regulatory regime, which actually has two definitions of fairness. One’s called disparate treatment and one’s called disparate impact. And disparate treatment is like, am I treating you differently because of who you are? So for many years, women were prohibited from getting credit cards unless they had the authorization of a man. They were being treated inherently differently because of who they are. That’s disparate treatment. Disparate impact is, do my decisions appear to be neutral, but in fact, are causing an unjustified adverse outcome on some particular community? And so the good news is that there are definitions of fairness in financial services and there are statistical or mathematical ways that you can try to answer those questions.
[05:21] Vince Passione: I think I know the answer to this, but first is, look, we had all these regs to make sure that we were being fair when we were doing it the old way, and we’ll talk about tech in a minute. I want to dive into the old method of linear regression testing. And now AI comes along, and the whole thought of AI is, well, it’s going to solve the problem. It’s going to eliminate bias. It has this ability to deal with all these attributes. So what went wrong?
[05:43] Kareem Saleh: Well, it’s more about AI has this tendency to overfit its decisions on populations in the data that are well represented. Populations in the data whose information is present and correct. And the problem of course is that for all kinds of historical reasons, there are many populations for whom the data is messy, missing or wrong. And the good news about AI is that it can consume all that data that’s messy, missing or wrong, where the traditional methodologies couldn’t. But in consuming all that information, it has a natural tendency to overfit its decision-making criteria to the populations that it understands, that are easy to score. But that means that there are a lot of populations which are hard to score. And if you don’t correct for the natural tendency that AI has, the models or the algorithms, the AIs, won’t spend much time trying to understand how to underwrite these harder-to-score populations. And so that’s fundamentally the risk, that for populations that are hard to score, whose data is more likely to be messy, missing or wrong, the AIs will systemically rate them as riskier.
[07:13] Vince Passione: So we’re going to get into why, what… And you’re using AI to determine whether those models have bias in them. And I want to get back to that for a minute, because it seems like there may be an interesting sort of loop in that logic.
[07:28] Kareem Saleh: We joke that AI is both the problem and the solution.
[07:31] Vince Passione: Exactly. That’s what I was thinking about as I started listening to some of your prior podcasts. But let’s step back. Let’s talk about the history around technology that was used to make these kinds of decisions. So I’ve been around financial services for over 40 years. I’ve been in lending for probably 30 of those years. And my entire career in lending, we used what I would call the old-fashioned historical model of credit scoring where we used linear regression models around a dependent variable like probability that you’ll pay, and we looked at independent variables like your income or your payments or your other loans. So talk to my listeners about that, versus, well, AI was going to fix it, how does it differ? And then I want to go back to this discussion about this interesting problem of AI as a solution and probably also the problem.
[08:27] Kareem Saleh: Yeah, so you’re right that it’s not like we’re coming to these issues of fairness and predictive models without the benefit of 30 plus years of financial institutions trying to comply with the Equal Credit Opportunity Act and the Fair Housing Act and other laws that prohibit discrimination in a range of domains. But the old logistic regression model world, logistic regression models kind of assume linear relationships, but it turns out that a lot of credit behaviors are non-linear. And in order to capture what’s really going on in a machine learning model requires a much more sophisticated analysis than what’s required to capture what’s going on in a logistic regression model. Logistic regression model, you can kind of just look at the coefficients on the variables and understand, okay, my model takes this variable heavily into account or that variable heavily into account. But the problem is that sometimes seemingly fair variables will interact in ways to encode information that the machine can see but that no human can see.
[09:45] Kareem Saleh: And so what we find is when you are now transitioning to this era of big data, increasingly… I will call them black box, although many of them are also increasingly explainable models, and layer on top of that a choppy credit environment where lenders are constantly adjusting their approval rates to account for things like the Federal Reserve raising interest rates three times in 2022, or inflation. And so in a world of big data, artificial intelligence and an uncertain macroeconomic environment, you can’t do a kind of low rigor, infrequent fair lending analysis, which is what I would argue was kind of done in the old way. It was kind of done annually, done retrospectively and done kind of on this univariate basis. But we’re headed for a world of kind of continuous underwriting with all kinds of data inputs. And you can’t basically have underwriting for the AI age and compliance from the Stone Age. You’ve got to be as sophisticated in your governance of these models as the systems are.
[11:12] Vince Passione: So let’s go back to maybe just take an example. So one of my clients were lending actively in 2022 to consumers. And the stimulus checks that went out caused those individuals to suddenly have this windfall in their checking account. And many of those models, traditional models picked that up, and he went from potentially having a FICO score of, I don’t know, 680 to perhaps maybe 720, 730. So I think most of my clients would understand that the historical way of underwriting that particular customer probably got you in trouble. Now, talk about how AI would’ve caught that. And then I want you to talk a little bit about, now, on the buyer side, if I was a thin file customer, how would your algorithm pick that up and say, “Look, this is what you’re doing, this is why”?
[12:08] Kareem Saleh: Basically the answer to all of that is very robust monitoring. Monitoring the distribution of the inputs to the system, monitoring the distribution of the outputs to the system, monitoring the fairness outcomes of the system so that you have essentially 24/7, 365 visibility into who’s walking through the door, are the decisions about them being made reasonably? Has there been any shift either in the macroeconomic environment or in the distribution of people that the model is approving? And what are the model fairness trends? That’s a thing that we’re doing a lot these days for folks, is monitoring the fairness outcomes so you can get ahead of a regulatory risk instead of the old way where you wait a year and then look back retrospectively to see if you had a problem.
[13:08] Jim Merrill: This is Jim Merrill, President and CEO of Inspire Federal Credit Union. For the last 13 years, we’ve partnered with LendKey to elevate our lending services and strengthen our commitment to providing the best financial solutions for our members. The team at LendKey is not only knowledgeable and responsive, but also genuinely committed to our success. They have empowered us to better serve our members and have been a true partner, not just another vendor.
[13:39] Vince Passione: So let’s move to… We’re going to talk about tech some more, but I want to move to the data furnishing side of the credit reporting business, because we had Christian Widhalm on, he’s a LendKey alum, but now he runs a firm called Bloom Credit. And they built a business called data furnishing as a service where they’re helping fintechs who couldn’t figure out how to furnish properly and were making mistakes, had to improve that process and take some of the noise out of poor data furnishing, which affects consumers bureaus. But his comment was more around the rails today for reporting aren’t set up to take on a lot of the data, alternative data, that we probably need to consume in order to get those AI models to work well. And you talk about these seemingly disconnected variables. So what are some examples of those variables? And do you agree with Christian that the current rails for furnishing probably don’t support reporting those variables back to the bureaus?
[14:40] Kareem Saleh: So on variable interactions, we see them all the time. And if you think about it, there are all kinds of ways in which variables interact in your life and you may not realize it. So let me give you a few examples. Let us say for a moment that we were trying to build a model that predicts the sex of an individual. It’s a terrible idea, we would never do that in financial services, it’s illegal, I’m only trying to make a point. And what if I told you that as an input to that model, I was going to give you height? Well, you’d say, “Height is somewhat predictive of sex because men tend to be taller than women, but of course height is not perfectly predictive of sex because there are some really tall women in the world and there’s some really short men in the world.” So what if I told you, “Okay, in addition to height, I’m going to give you weight,” because even at the same height, men tend to be heavier than women due to things like bone muscle density and testosterone.
[15:51] Kareem Saleh: But of course the problem with the model that seeks to predict sex on the basis of height and weight is that it’s going to misclassify every child as a woman. So what if I told you, “Okay, I’m going to give you birthdate to control for the fact that there are children in the world.” Well, now our system, our model of predicting sex, is looking pretty good. But if I told you a moment ago that birthdate was predictive of sex, you would’ve told me I was crazy. But this is just an example of how these seemingly fair, seemingly independent variables interact in predictive models to encode information that we could never imagine was there. And so the challenge is, how do you maximize the predictive power of that information, but minimize the disparity driving effect it’s having on these groups?
[16:49] Vince Passione: So those are incremental variables, and we’re talking about data furnishing. So how do you now take that and can you furnish that?
[16:58] Kareem Saleh: It’s really hard. Your colleague who was on and told… I mean, we produce an amount of data exhaust that is an order of magnitude bigger than what was conceived when the credit furnishing infrastructure was built. So it’s hard to report this stuff back. I know that the bureaus are working on it, and I think Metro 2 reporting is going to improve some of that, but it’s hard.
[17:28] Vince Passione: So you touched on gender in one of your examples, and you wrote an article that was fairness through awareness and really discussing the need for demographic data. And I think you said it’s time we start using race and gender to combat bias, which seems to be contradicting itself. So didn’t we build these algorithms so that… I mean, I have clients today that will not take a picture, an ID, before they make the credit decision for fear of somehow biasing the decision. So explain that, Kareem.
[17:57] Kareem Saleh: Yeah, so this idea that… As I just explained to you through, the ways in which these seemingly fair variables actually encode information, this idea that we’re not using it because it’s not explicitly on the list of stuff we’re using, that’s just like a story we tell ourselves to feel good at night, to sleep at night. We are using it, it’s just encoded in other pieces of information. I don’t think anyone is saying use it directly, because that’s illegal. So the whole question is just like, how do you use it, at what stage do you use it, et cetera? And Zest has a technique and Upstart has a technique, and I actually, to be perfectly honest with you, don’t think any of the techniques… We have a technique, I talked about the two techniques that we use, and while I am quite proud of them, and my name is on the patents and I’m really excited about that, I’d never invented anything before. I also think that the techniques don’t really matter very much. I think what matters is given a technique and a range of options, how do you pick?
[19:19] Kareem Saleh: And there, I do think we’ve actually done the best work, because we’ve created this stress testing methodology, which allows you to compare how two models are going to perform, both from an accuracy perspective and from a fairness perspective under many different through the door populations, and at many different credit policies and approval rates. And that allows you to objectively stack rank your options in a world where you can generate hundreds or thousands of alternative models.
[19:53] Kareem Saleh: And so the question is like, well, I don’t think any of us think you should use it as a direct input to your decisioning, that has all kinds of legal and ethical problems with it. But when you’re building the model, maybe you should understand that women may sometimes have different credit characteristics than men, and maybe we should set the weights on the variables in ways that accommodate or that treat everybody fairly. I’m not suggesting that we reduce the accuracy of the decision. I’m saying that, let’s be as accurate as we possibly can, but also correct for this tendency to overfit our criteria for making decisions to the population that is majority represented in the data set we’re working with.
[20:44] Vince Passione: That feels like a slippery slope. When you go to a regulator, you go to the CFPB and say, “We’re going to use race and gender because we believe that in the historical data, the bias is there even though you didn’t realize it, so you might as well identify it and then refit the model. It seems like a slippery slope. How does the regulator react to that?
[21:04] Kareem Saleh: Believe it or not, the law actually requires it. It’s just that there were no good tools for doing this before. So remember earlier at the top of the call, we were talking about the different definitions of fairness, and we talked about disparate treatment and we talked about disparate impact? Well, the disparate impact test says you can’t discriminate unless it’s in furtherance of a legitimate business objective, and there’s no less discriminatory method of achieving that business objective. And that third prong, that third piece of that test, the less discriminatory alternative piece, there weren’t really good tools to search for less discriminatory alternatives. So the common fair lending practice was to say, “Well, yeah, I might approve this or that protected group at lower rates, but look, it’s justified by the riskiness of that population,” and that was more or less enough to get your regulator to go away.
[22:07] Kareem Saleh: But increasingly, and I think this is the thing that is important for your audience to know and understand, in a world of AI where it’s possible to generate many different variants of your algorithm, many of which will perform effectively the same from an accuracy perspective, but vary widely on other dimensions you might care about like fairness, robustness, stability, et cetera, the regulators are increasingly saying, “Hey, those business justifications aren’t good enough. Show me that you looked for a way to be fairer within your risk tolerance.”
[22:44] Vince Passione: So when we go out and we look at learning data and how to get it, are there privacy implications about this? And there’s lots of changes, 1033 in the consumer and their privacy, and how they can direct someone who can look at their information or share their bank information with another application. How do you feel about the privacy implications of it?
[23:05] Kareem Saleh: Ultimately, privacy is one of the most difficult values that we need to preserve in the AI era, and I think these are decisions that have to be made for people by themselves. But I encourage everybody to think about whether there’s some way of sharing their demographic information, perhaps in an anonymized way or through the census in a way that allows you to be counted, because on balance, I think being counted makes it more likely that your community will be better served by the financial industry over time because you’ll be underwritten to standards that are appropriate for you.
[23:40] Vince Passione: Thanks so much for joining me. Appreciate your time and your insights. They were great. Thanks to our listeners for tuning in, and don’t forget to subscribe so you can hear more of our episodes. And I’ll meet you back here at our next 22 Minutes in Lending. Kareem, thanks again.